In the bestseller White Fragility: Why It’s So Hard for White People to Talk About Racism, Robin DiAngelo writes, “Prejudice always manifests itself in action because the way I see the world drives my actions in the world. Everyone has prejudice, and everyone discriminates.” She later clarifies that White supremacist messages “circulate 24-7 and have little or nothing to do with intentions, awareness, or agreement.”
Work such as DiAngelo’s—suggesting a universal, unconscious anti-Black bias among White people that always manifests in action—has inspired a multibillion-dollar Diversity, Equity, and Inclusion (DEI) industry that profits off of exorbitantly expensive anti-racism workshops. DiAngelo herself charges between $10,000 and $15,000 for a few hours of training.
But beyond the cost, the methods employed by “diversity trainers” like DiAngelo are at best questionable, and at worst perpetuate dehumanizing stereotypes.
At Evergreen State College, one DEI workshop facilitator asked participants to imagine they were in a canoe, shoved around by violent waves and bombarded by fierce headwinds, as it journeys toward equity. The faculty organized themselves into the physical shape of a canoe and the facilitator made senior administrators ask for permission to board while committing to furthering DEI, all as the sound of crashing waves blasted through the room. Senior administrators committed not by proposing new institutional policies, but by professing agreement with the importance of DEI.
A private theater company hosted a different training where participants were instructed to recall past interracial interactions and list microagressions they were assumed to have perpetrated. One participant thought back to when they passed a Black man wearing a suit in the hallway and playfully called to him, “looking sharp.” Suddenly, they wondered if they would have said the same to a White man. Participants were also asked to form racially segregated “affinity groups” so that the White staff could confront their racism without harming their minority peers.
Three public agencies in Seattle implemented racially segregated DEI trainings to help White employees “accept responsibility for their own racism.” There was no evidence provided of any White employee’s racism because the trainings were inspired by widespread “institutional” or “internalized” racism.
Sandia National Laboratories, a research laboratory that specializes in nuclear weaponry, sent its White male administrators to a luxury resort for mandatory DEI training. Facilitators made the participants list White male culture’s negative elements. The list included “white supremacists,” “KKK,” “Aryan nation,” “MAGA hat,” “privileged,” and “mass killings.” They were then made to condemn the roots of White male culture, such as “rugged individualism,” “a can-do attitude,” “hard work,” and “striving towards success” that are “devastating” to women and people of color.
These trainings almost always fail to reduce biases, and the key presumption underlying each of them—that all White people harbor implicit biases that manifest in behavior—is wrong. Research shows that implicit or unconscious bias has no meaningful relationship with actions, and that measures of implicit bias are effectively meaningless.
Understanding Implicit Bias
It is well-established that implicit bias exists. Psychologists know most mental processing occurs subconsciously and that information and associations can be encoded in memory without a person’s knowledge or agreement.
Psychologists most commonly measure implicit bias with the Implicit Association Test (IAT). During an IAT, test-takers are shown a series of White faces, Black faces, positive words, and negative words, which they categorize using the “E” and “I” keys. For instance:
Press E | Press I | |
Round 1 | African American | European American |
Round 2 | Bad | Good |
Round 3 | Bad or African American | Good or European American |
Round 4 | Bad or African American | Good or European American |
Round 5 | European American | African American |
Round 6 | Bad or European American | Good or African American |
Round 7 | Bad or European American | Good or African American |
The IAT claims to measure people’s automatic racial preferences by comparing response latencies between rounds. If a test-taker is quicker to associate “Bad” with “Black people” in rounds three and four than they are to associate “Bad” with “White People” in rounds five and six, this is taken as evidence of their implicit anti-Black bias.
Implicit Inconsistencies
Because the IAT measures implicit bias in this indirect way, its measurements are influenced by a variety of extraneous factors and vary wildly. Re-test results are often completely different from those of other implicit bias tests and align with initial IAT results only about 56 percent of the time.
Test-takers are also predisposed toward bias by the administration of the test itself. IATs report bias favoring and opposing random objects simply by changing their color and size. Ironically, the data transformations implemented to correct this predisposition further predispose results toward bias.
Some test-takers are even more biased in favor of higher prejudice scores because they take longer to process unfamiliar information. When people take IATs measuring bias toward nonsocial categories—“flowers” and “insects”—they respond quickly to the familiar association of “flowers” with “pleasant” and “insects” with “unpleasant.” Conversely, they respond slowly to the unfamiliar association of “flowers” with “unpleasant” and “insects” with “pleasant.” It’s possible that familiarity with social issues—like the concept that American culture views Black Americans negatively—and the time it takes to comprehend unfamiliar information, impacts IAT results.
IAT-measured bias can also be reversed by changing the social context of images and words presented during testing. Racial bias IATs report that when White test-takers are shown pictures of Black faces in a factory, prison, and church, they exhibit an anti-White bias, a pro-White bias, and no bias, respectively.
Similarly, White test-takers’ pro-White bias is significantly reduced by exposing test-takers to admired Black and disliked White faces before the test. Even changing the shirt of the IAT administrator reduces bias.
The ability of small environmental variations to sway IAT results is one reason why implicit bias—as measured by the IAT—has no meaningful connection to behavior.
One analysis of forty-six published and unpublished studies found that the IAT was not related to peoples’ actions. Two additional meta-analyses agree.
While other studies have claimed IAT scores do predict behavior, they are often rife with dishonest statistics and overstated conclusions that mask their true findings.
Dishonest Statistics
Scientists use statistical standards to determine if an observed relationship is “significant” and not due to chance. Significance is represented by a p-value; the lower the p-value, the higher the significance. There are also asterisk notations used to denote different levels of significance.
Asterisk Notation | p-value | Meaning |
n.s. | p>=0.05 | Insignificant |
* | p<0.05 | Significant |
** | p<0.01 | Very significant |
*** | p<0.001 | Extremely significant |
Some IAT studies manipulate their significance threshold or the meaning of notations to exaggerate their findings. Take Rachlinski et al. and Green at al., for instance. Rachlinski examined how IAT scores related to judges’ sentencing determinations and predictions of recidivism. Green et al. looked at how IAT scores correlated with physicians’ likelihood to diagnose coronary artery disease, as well as prescribe treatment.
Rachlinski et al. conclude that “implicit biases can affect judges’ judgment” based on their finding that IAT scores had a “marginally significant influence” on sentencing. But it turns out that IAT scores aren’t significantly related to sentencing at all. Rather than using the standard 0.05 threshold for significance, Rachlinski et al. used 0.1. This detail—which allowed them to label a p-value of 0.07 as “significant”—is not mentioned in their methods and materials section, but implied in footnote ninety-four.
Using the standard significance threshold renders their finding insignificant. And ironically, despite manipulating the significance threshold, Rachlinski et al. still failed to find a significant relationship between judges’ IAT scores and predicted recidivism.
Green et al. misleadingly alter scientific notation to imply significance when it doesn’t exist, then contradict themselves about the significance of their key finding. They use “**” to indicate 0.05<p<0.11, not p<0.01, and state that one figure reports a significant relationship while the figure itself states the opposite. They also don’t include error bars on their figures, hiding uncertainty in their data.
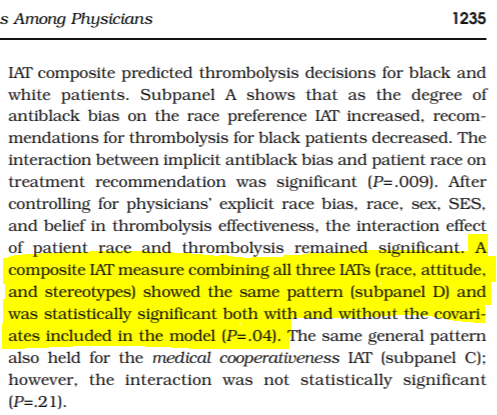

The misrepresentation of statistics in IAT research is not an isolated phenomenon. Many recent studies have reviewed findings from previously published papers and found their conclusions to be unsupported.
One study by Blanton et al. reexamined two influential papers that claimed to show a relationship between IAT results and discriminatory behavior.
For the first, not only did reanalysis reveal significant methodological manipulations, but also showed that many who received a pro-White bias rating from the IAT exhibited a pro-Black bias in their behavior. Surprisingly, those with IAT scores indicating severe implicit bias “were the least behaviorally biased in the sample.”
For the second, reanalysis showed statistical significance to evaporate when “traditional standard errors”—that correct for weakly correlated findings—were included in the analysis, as is done in honest procedures. Likewise, the removal of outliers abolished significance regarding the relationship between IAT scores and behavior. In fact, reanalysis found that 95 percent of changes in behavior were unrelated to IAT scores.
Another reexamination took data from Hoffman et al. and reversed their analysis. They found that even when participants behaved neutrally (exhibited no bias), they received an IAT score indicative of a moderate pro-White bias. Correcting for this baseline skew obliterated Hoffman et al.’s conclusion that IAT predicts behavior. Surveying other studies uncovered similar IAT skew with similar implications for their findings.
Overstated Conclusions
IAT proponents often make grandiose claims based on shockingly weak findings. Worse, some purposefully make these claims in their papers’ abstracts, where lay-readers and reporters—who are unlikely to delve into the data or understand how to interpret results—will see them.
Statistical significance is a measure of scientists’ confidence in their observations. It says nothing about the magnitude of a finding.
One statistic often used to represent magnitude is r2, or rr. You can learn how and why r2 is calculated here, but all that really matters is knowing how to interpret it; an r2 value represents the percent change in a variable that is explainable by changes in a different variable.
Imagine scientists gave ten plants increasing amounts of fertilizer and measured their growth after one month. Then, they calculated an r2 of 0.9, meaning 90 percent of plant growth is explainable by the amount of fertilizer given to each plant.
“Is explainable by” is the key phrase here. The r2 value is the maximum change in one variable that can be explained by another. High r2 values can be observed even though the variables are obviously unrelated. There is an entire website of such spurious correlations that shows the dangers of lending too much credence to an r2.
In implicit bias literature, scientists focus much more on the statistical significance of their findings than on the magnitude of the relationships they uncover. This inflates the relevance of their observations.
Dovidio and Kawakami announced that implicit bias “significantly predicted” White peoples’ nonverbal friendliness toward Black people, as well as observers’ perceived bias in interracial interactions. Yet, their abstract is void of data. They actually found that implicit prejudice explains at most 17 percent of changes in nonverbal friendliness (r=0.41, r2=0.17) and at most 19 percent of changes in observers’ perceived bias (r=0.43, r2=0.19). They also found that implicit bias had nothing to do with verbal behavior.
Cameron et al. and Greenwald et al. are most direct about their results. Both abstracts contextualize their findings, albeit indirectly, by numerically acknowledging that implicit bias explains at most 8 percent of behavior (both studies found that r=~0.28, r2=0.08).
Conversely, Kurdi et al. are most dishonest. They report correlations between the IAT and criterion behaviors—person perception, resource allocation, and nonverbal measures—but you need to sift through the depths of their paper and supplementary materials to find the correlation’s strength: a whopping 1 percent of changes in behavior are explainable by implicit bias (r=0.10, r2=0.01).
All of these studies concluded that implicit bias is significantly related to behavior—and some noted these conclusions in their abstracts—yet all of them found that the vast majority of behavior has nothing to do with implicit bias.
Study |
Conclusion |
Minimum % of Behavior Unrelated to Implicit Bias |
Dovidio and Kawakami (did not use IAT) |
“. . . the response latency measure significantly predicted Whites’ nonverbal friendliness and the extent to which . . . observers perceived bias in the participants’ friendliness.” (abstract) |
83% and 81% |
Cameron et al. (did not use IAT) |
“the authors found that sequential priming tasks were significantly associated with behavioral measures (r = .28)” (abstract) |
92% |
Greenwald et al. |
“This review justifies a recommendation to use IAT and self report measures jointly as predictors of behavior” (pg. 32) |
92% |
Kurdi et al. |
“. . . attitudes, stereotypes, and identity, measured both using self-report and less controllable responses such as the Implicit Association Test, are systematically related to behavior” (pg. 36) |
99% |
People Have Self-Control
Another reason why implicit bias is not meaningfully connected to behavior is that people have self-control—ideas pop into our minds all the time that we choose not to act on.
Every action we take is influenced by our motivations, opportunities to question the action, our ability to act, and awareness of our biases.
If you are motivated to act neutrally, if you have the opportunity to think through your actions, if you are capable of changing your actions, or if you are aware of your biases, then it is unlikely your actions will be driven by implicit bias. Fortunately, almost every real-world interaction meets at least one of these conditions, which means that almost every real-world interaction is unlikely to be driven by implicit bias.
This model of behavior is why many IAT proponents focus on correlations between IAT scores and nonverbal behaviors, such as blinking rate, amount of eye contact, and time spent smiling, fidgeting, or leaning toward a speaker. Pro-IAT researchers know that more conscious behaviors subject to deliberation are less likely to correlate with IAT scores and less likely to lead to politically relevant conclusions. Though, ironically, even the relationships between IAT scores and nonverbal behavior uncovered by studies are weak or outright non-existent.
Implicit Bias is a Useless Concept
There are two theories as to why IAT-measured implicit bias fails to predict behavior: either implicit bias has no meaningful relationship with behavior, or it does but it cannot be reliably measured. However, it doesn’t matter which theory is correct.
Accepting the first would be to acknowledge that implicit bias is meaningless and has no implications for behavior in the real world.
Accepting the second would be to reject the scientific process altogether. Notice that the second starts with the conclusion one may want to reach, “implicit bias does meaningfully influence behavior,” and then implies what actions they should take to do so. This is not how science is supposed to work. Science requires finding evidence before drawing conclusions.
Further, the second is unfalsifiable; if scientists can’t measure implicit bias, then the hypothesis that it influences behavior is neither provable nor disprovable. This is inherently unscientific.
Since the second acknowledges there is no measurable evidence that implicit bias impacts behavior, an honest scientist would fall back to their null hypothesis. In this case, the null hypothesis is the first, that implicit bias and behavior are unrelated.
No matter how you slice it, implicit bias is an effectively useless concept that fails to explain real-world phenomena.
Move on From Implicit Bias
DiAngelo closes out White Fragility insinuating that White people—to combat their implicit racism—must participate in the sort of DEI training that DiAngelo herself has profited from for years. “It is a messy, lifelong process, ” DiAngelo acknowledges, but one she believes is necessary to align White peoples’ professed values with their actions.
Ignoring the obvious grift here, there are no scientific justifications for DEI workshops. Based on the evidence we have now, implicit bias is just not meaningfully related to behavior.
However, there are lessons to be learned from the recent scientific focus on implicit bias. This focus reflects a broader cultural movement to recognize and explain America’s startling racial disparities. The average White family holds 6.7 times more wealth than the average Black family. Black entrepreneurs are significantly underrepresented among Fortune 500 CEOs, and the median White worker makes 28 percent more than the median Black worker. Black people are overrepresented in fatal police shootings and incarcerated in state prisons five times more often than White people.
As a country, it is important that we grapple with our inequities. We should ask ourselves difficult questions like, “If America presents equal opportunity to all, then why are there such startling divides by race?”
But pinpointing implicit bias as the cause of racial disparity is like an avid smoker blaming their lung cancer on not eating enough broccoli. Maybe eating broccoli would have helped reduce their risk, but by so little it wouldn’t have mattered. Likewise, maybe the complete abolition of all implicit biases would reduce racial disparities by a tiny fraction, but after all is over and done with, almost all of America’s disparities would persist.
We should move on from implicit bias. Focusing on a niche psychological theory that fails to explain the vast majority of behavior will not make Black and Brown peoples’ lives any better. Instead, we should debate policies that will actually correct historical injustices. Discussions about reparations or general anti-poverty initiatives are more fruitful than filling DiAngelo’s pocketbook.